Setting up a sweep job with grid sampling
Earlier in the chapter, we cloned our sample notebook to leverage this material. The notebook for this chapter, '
Chapter 4
- Hyperparameter Tuning'
, provides a review on creating a job command to create a logistic regression model by leveraging an sklearn
pipeline and mlflow
capabilities.
The code is then updated and placed into a new directory – the hyperparametertune
folder. The code leverages python’s argparse
module, which enables you to pass parameters into scripts. To run the script that has been generated by this notebook, we will create a job command and update the job command to include the hyperparameters as shown in the following code snippet:
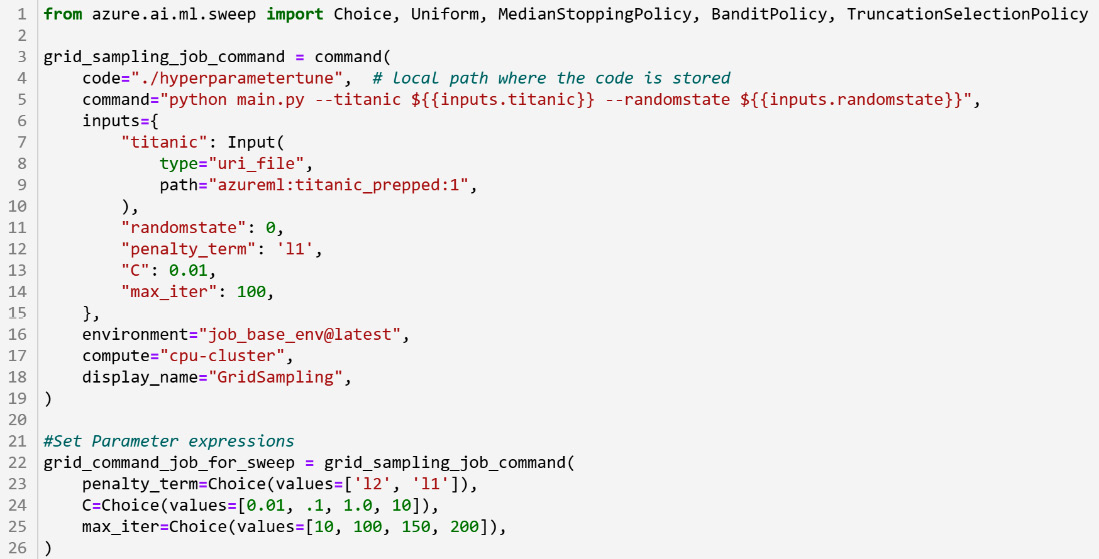
Figure 4.16 – Job sweep command with hyperparameters for grid sampling
Note that the hyperparameters have been included as inputs to the command, but their values are added to the command in line 22 in the preceding figure. This...