To explain the Bellman equation, it's better to go a bit abstract. Don't be afraid, I'll provide the concrete examples later to support your intuition! Let's start with a deterministic case, when all our actions have a 100% guaranteed outcome. Imagine that our agent observes state and has N available actions. Every action leads to another state,
, with a respective reward,
. Also assume that we know the values,
, of all states connected to the state
. What will be the best course of action that the agent can take in such a state?
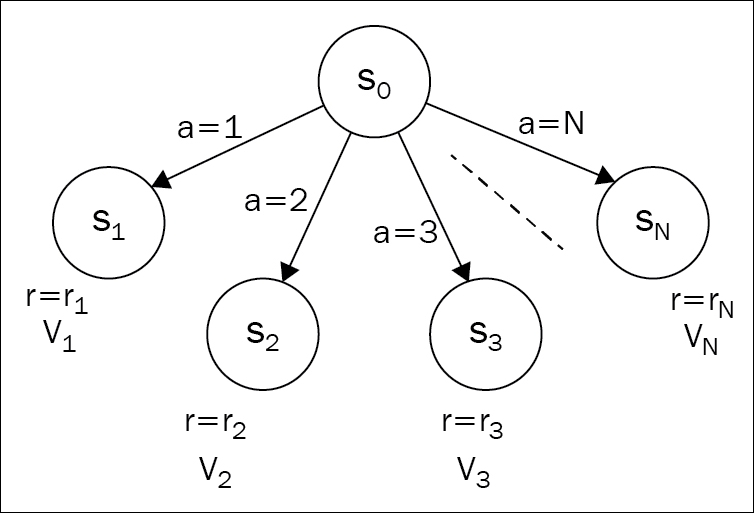
Figure 3: An abstract environment with N states reachable from the initial state
If we choose the concrete action , and calculate the value given to this action, then the value will be
. So, to choose the best possible action, the agent needs to calculate the resulting values for every action and choose the maximum possible outcome. In other words:
. If we're using discount factor
, we need to multiply the value of the next state...