Scaling for big data packages
In this section, we will look at different tools that will help us with scaling the ETL pipelines for big data packages.
Dask
When faced with processing capacity contingencies, it makes sense to increase your capacity with the assistance of additional devices. This is the premise for the parallelization of tasks in Python. Similar to batch processing, partitioning data (aka separating the data into equal, bite-sized bits), allows large data sources to be processed in identical, synchronous ways across multiple systems.
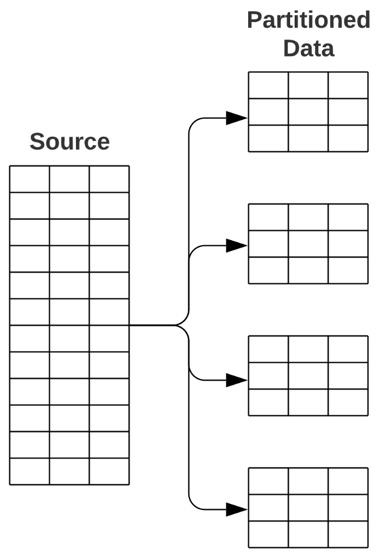
Figure 3.5: Concept of partitioning data
Dask is the Python library that allows for the parallelization of processing tasks in a flexible and dynamic scope. Dask is a cloud-based module that uses “clusters”, which are extra processing units in the cloud on standby, that can lend a helping hand to heavy-processing tasks initiated on your local device. The creators of Dask designed this parallelization...